Artificial Intelligence
Artificial Intelligence
S tone Soup Tech is an Artificial Intelligence oriented software and consultancy agency focusing on providing strategic research and development. Our AI department is headed by:
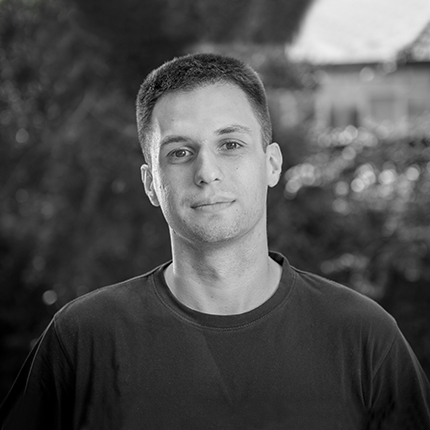
Valentin Lungu
lecturer at the Polytechnic University of Bucharest teaching Machine Learning applications
member of the Romanian Association for AI (ARIA)
member of the AIMAS-WO promotion and development team
former research developer at the Serious Games Institute (Coventry, UK)
former member of the Laboratoire d’Informatique de Paris 6
Expertise
Game AI, Machine Learning, Expert Systems, Planning, Knowledge Representation and Reasoning
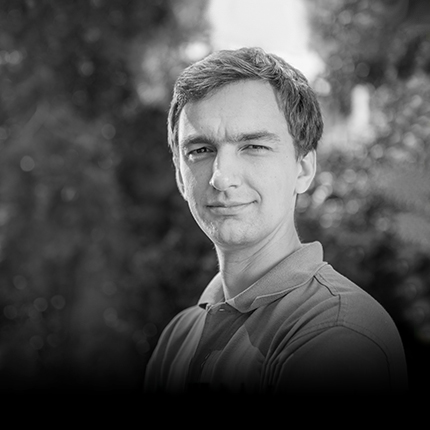
Andrei Olariu
ranked one of the top 100 data scientist on Kaggle.com
worked on terabyte-scale datasets at Twitter
TA at the University of Bucharest, teaching Neural Networks and Algorithms and Data Structures
Expertise
Predictive Analysis, Natural Language Processing, Machine Learning
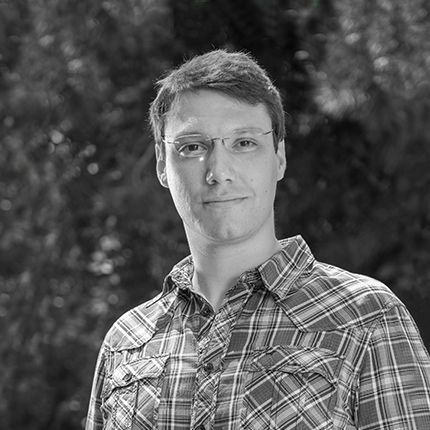
Brad Constantinescu
designed & implemented multiple AI centric applications including statistical classifier for signal auditing lead generation, various recommendation systems, AI powered social bots
former member of the AI-MAS WO Team, implementing a platform for Game AI competitions
Expertise
Natural Language Processing, Statistical Classifiers, Behaviour Analysis, Machine Learning
It is a system that combines Business Intelligence with Health education. It improves the health-care environment through smart online education and actionable insights offered to the specific entities by making use of Artificial Intelligence and the power of the Cloud.
Our system integrates artificial intelligence (AI) technology and internet-based health information to deliver a system that indexes, analyses, and feeds health-related articles to users based on individual reading preferences.
Our first generation system has 75 indexed websites with approximately one million articles within our system on various health related topics. We can analyze data on a particular disease, a drug, or a symptom and provide data summaries. These can be used by pharmaceutical or health-care consumer companies in the pre-discovery phase of drug/product development when understanding all facets of a disease or disorder are critical before embarking on further drug and or product development. Both pharma and consumer companies can capitalize on a detailed understanding of consumer trends. Analyzing our user base for patterns and trends in most frequently read articles, most shared and most commented on articles can provide valuable information on future market trends. This information is otherwise difficult to capture and our system provides a unique opportunity for pharma and consumer-based companies to gain a better understanding of their costumer.
This system uses Artificial Intelligence to provide fast insights and drive agile, competitive decisions and actions for the real estate businesses, while helping regular users find what they need faster by using analysing to their behaviour and preferences.
Our solution brings Artificial Intelligence into play in the online and mobile real estate market. It harvests data from the web as well as user behaviour from mobile apps. Combining these two data sources with data mining solutions and machine learning techniques, we can provide valuable insights and analytics, as well as uncapturable information for the real estate industry. Our intelligent system recommends the right listings to users within minutes of utilizing our applications.